This is the multi-page printable view of this section. Click here to print.
Blog
Incremetal update
When it comes to incremental updates, the two previous approaches seem to take completely opposite paths.
GraphRAG uses a three-layer Leiden algorithm to abstract the original homogeneous knowledge graph across multiple levels. One major downside of the Leiden algorithm is that adding even a few new nodes can totally disrupt the existing community structure. In other words, trying to update the graph can force you to recompute an entirely new set of communities and summaries at all three levels. Sure, there are ways to mitigate this issue, but they often add significant complexity—and waste precious compute resources.
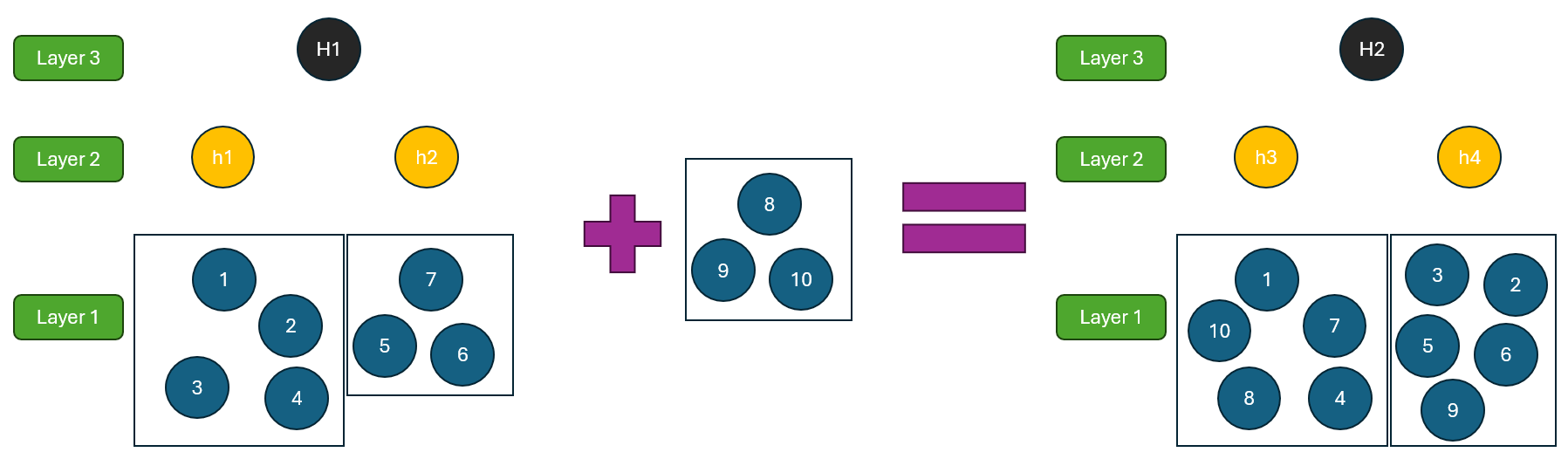
Figure 1. GraphRAG’s community structure — powerful, but hard to update efficiently
On the other hand, LightRAG takes a much simpler approach. It skips the whole graph summary idea and instead relies on paragraph-level structure, using extracted keywords as retrieval indices. This drastically cuts down on processing time and complexity—but it also means losing the rich corpus-level information enhancement that GraphRAG provides.
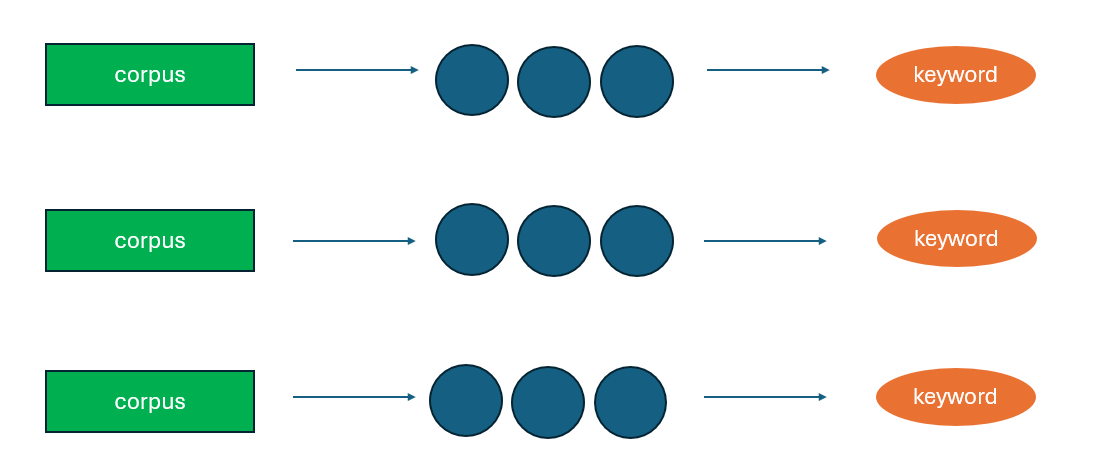
Figure 2. LightRAG simplifies things by relying on keywords, but sacrifices context-rich graph summaries
NodeRAG aims to strike a balance by combining the strengths of both approaches, leveraging the power of heterogeneous graphs. First, it uses only one layer of the Leiden algorithm to identify high-level information within communities—avoiding the three-layer complexity altogether. Second, thanks to its shallow PPR algorithm on heterogeneous graphs, new nodes can be directly merged into the existing graph. This ensures that semantically similar high-dimensional nodes remain close in graph space, while shallow PPR helps surface the most relevant nodes during retrieval.
Let’s walk through an example. Suppose we have a community centered around Concept A (represented as a high-level node connected to lower-level nodes in the heterogeneous graph). This community was built when indexing the original corpus. Now, we add new data. NodeRAG builds a new heterogeneous graph for the fresh corpus—say it forms a new Concept B community. NodeRAG then automatically merges the new and old graphs. Since some lower-level nodes are shared, Concept A’s and Concept B’s communities become interlinked. During retrieval, when a user query activates nodes under Concept A, the shallow PPR algorithm propagates through the graph, potentially reaching and highlighting Concept B—especially if some of its nodes were also relevant entry points.
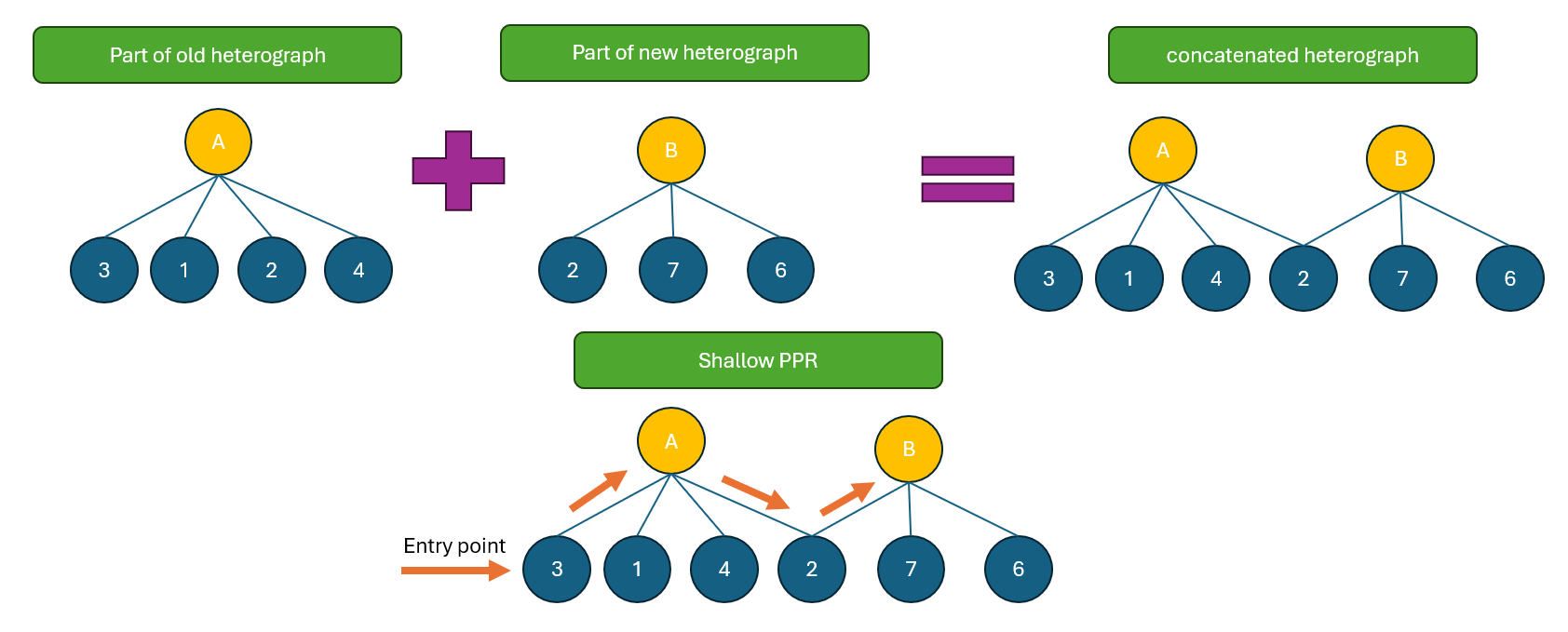
Figure 3. NodeRAG merges new and old graphs seamlessly, enabling efficient and context-aware updates
Structure Output
Structured Output Challenges in NodeRAG
NodeRAG relies heavily on breaking down long-form text into structured prompts for further processing. Specifically, it depends on the ability to consistently generate structured JSON outputs from longer text inputs. On the one hand, we need the system to maintain proper formatting even in complex, extended tasks. To achieve this, our project initially leaned on OpenAI’s structured output feature (docs here). With this, the model reliably broke down text blocks into heterogeneous nodes, which made downstream processing smooth and efficient.
However, as we looked to expand to other models, we hit some roadblocks. For example, OpenAI’s structural output failures (i.e., when the output didn’t follow the expected JSON format) occurred less than 1% of the time—pretty solid. But when we tried Gemini 2.0 Flash, this failure rate jumped to about 20%. Even worse, with DeepSeek’s official JSON output, nearly 60% of the API responses ignored the prescribed format, making error handling virtually impossible.
Because of these issues, in version v0.1.0, we only supported OpenAI and Gemini for structured output tasks.
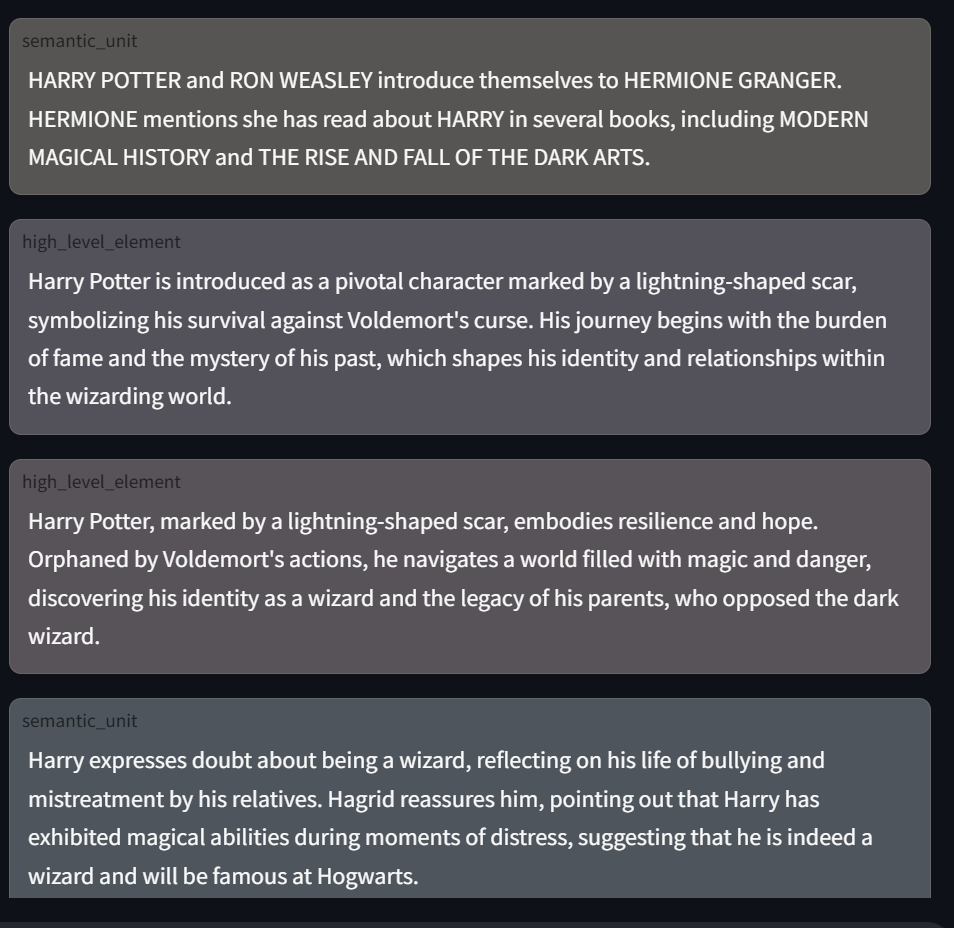
Figure 1. OpenAI GPT-4o-mini — highly reliable JSON output
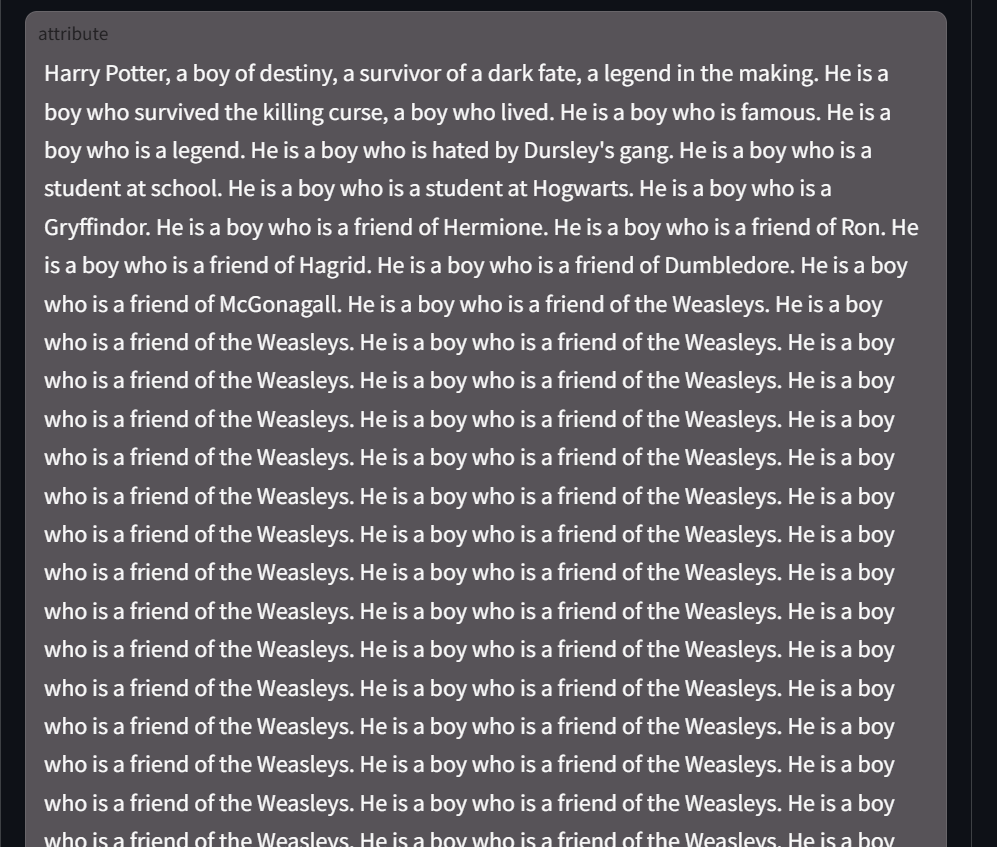
Figure 2. Gemini 2.0 Flash — acceptable format, but lower content quality
That said, even though Gemini managed to stick to the format more often than DeepSeek, we noticed issues with the quality of its outputs—such as repeated phrases, extra spaces, and generally lower fidelity content compared to OpenAI’s GPT-4o-mini.
Our Recommendation
So far, we recommend using OpenAI’s models for NodeRAG, especially for tasks that require consistent structured outputs. We’ll continue evaluating other models for their structured output capabilities to see if they can eventually fit into the NodeRAG pipeline.
Looking Ahead
One future direction we’re excited about: fine-tuning a lightweight model specifically for the NodeRAG text-to-node decomposition step. This task is essentially a blend of summarization and paraphrasing, so it’s relatively straightforward. The challenge lies in maintaining stable, structured outputs over long text inputs.
Once the text has been broken down into structured nodes, we can use more powerful models to extract higher-level insights and viewpoint summarization from the graph.